Elevating Cities: Exploring Skyscrapers and Urban Skylines Worldwide
Total images | : 13,488 |
Type | : organic |
Category | : Scenes |
Resolution | : Up to 1024px |
Storage size | : Up to 3 Gb |
File format | : JPEG |
The Urban Skyscrapers And Skyline Heights dataset is a meticulously compiled collection that offers a profound insight into the world's most iconic skyscrapers and the evolving urban skyline heights that define modern cityscapes. It serves as an invaluable resource for professionals, researchers, and enthusiasts intrigued by the architectural marvels that shape our urban environments.
Leveraging the power of AI and machine learning, this dataset opens up new avenues for analysis and understanding. By employing advanced algorithms, researchers can detect hidden patterns in skyscraper design, construction trends, and their influence on skyline compositions. Machine learning models can be trained to predict future skyline developments based on historical data, aiding urban planners in making informed decisions about city growth and infrastructure requirements.
The Urban Skyscrapers And Skyline Heights dataset, enhanced by AI and machine learning capabilities, transcends traditional urban studies, offering dynamic insights and predictive capabilities that revolutionize how we approach architecture, urban planning, and the sustainable development of our cities.
1. Skyscraper Design Optimization: Machine learning algorithms can assist architects and engineers in optimizing skyscraper designs by analyzing a vast array of parameters, including structural stability, material usage, energy efficiency, and aesthetics. This can lead to more innovative and sustainable designs.
2. Skyline Evolution Forecasting: AI models can forecast the evolution of urban skylines by considering factors such as population growth, economic trends, and architectural preferences. These predictions can aid city planners in preparing for vertical expansion and infrastructure development.
3. Tourism and Marketing Insights: AI can analyze tourist and social media data to understand which skyscrapers and cityscapes attract the most attention. This information can guide tourism boards and marketers in creating targeted campaigns and enhancing visitor experiences.
4. Environmental Impact Assessment: Machine learning can assess the environmental impact of skyscrapers by analyzing energy consumption, waste generation, and carbon footprint. This data can inform sustainability initiatives and drive eco-friendly architectural choices.
5. Architectural Style Recognition: AI models can be trained to recognize and categorize different architectural styles within skyscraper designs. This can provide architects with inspiration from historical styles and contemporary innovations.
6. Smart City Integration: AI-powered analyses of skyscraper data can contribute to the development of smart cities by optimizing resource allocation, transportation networks, and urban services based on the vertical density created by skyscrapers.
Environment: Commercial stock
Angle: Random
Augmentation: None
AR: Various
ACCURACY
This dataset contains a tolerance margin of 5% to 10% of associated images which might not reflect 100% accuracy in the metadata or image. As example for the error margin: an architectural drawing can appear due to its association with skyscraper construction. All metadata in this dataset had been created manually and might contain a low margin of error. The maximum resolution of each image might vary.
128px
$89
256px
$259
512px
$359
1024px
$459
Sample images in this dataset
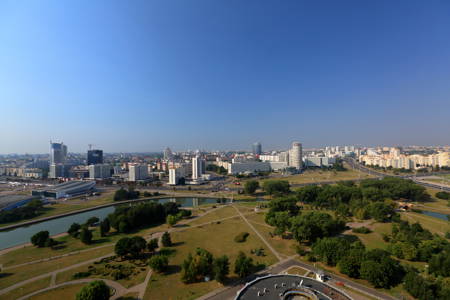
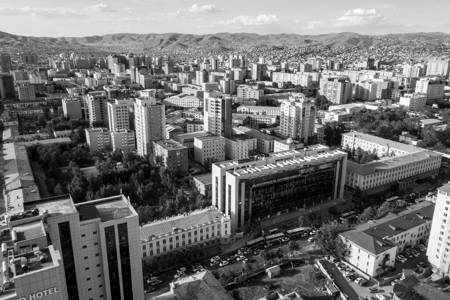
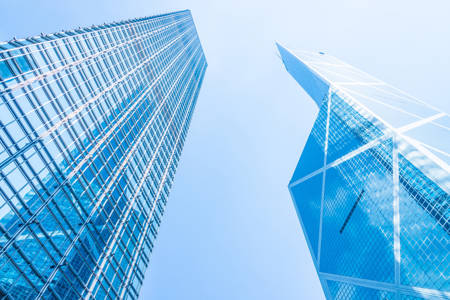
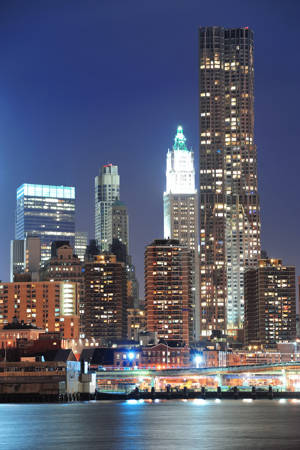
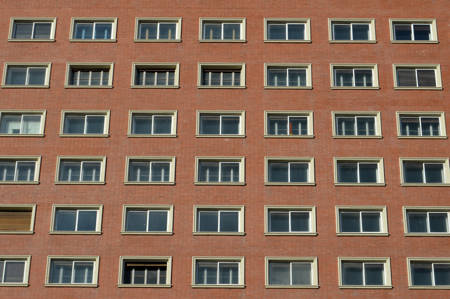
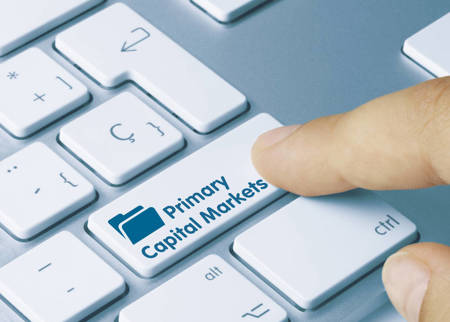
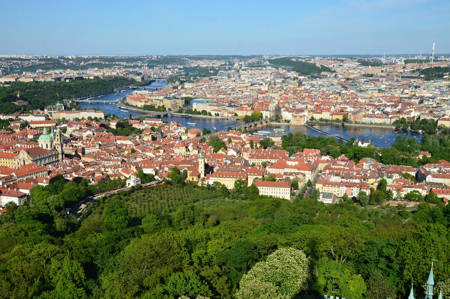
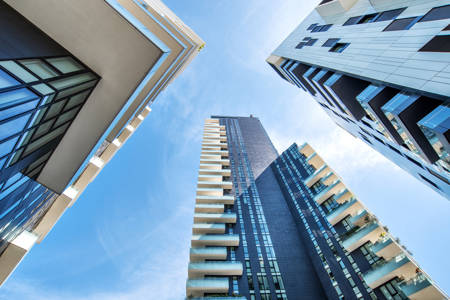
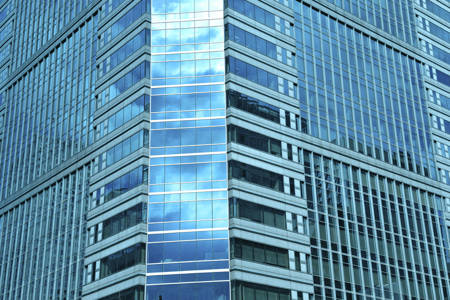
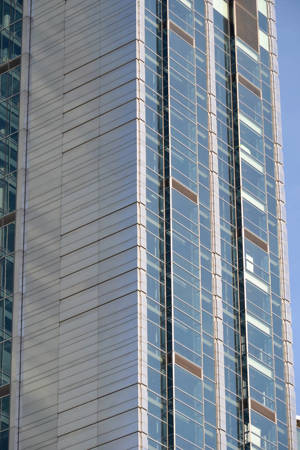
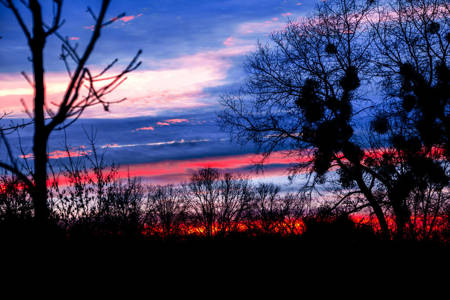
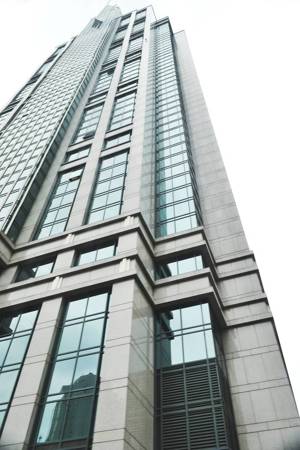
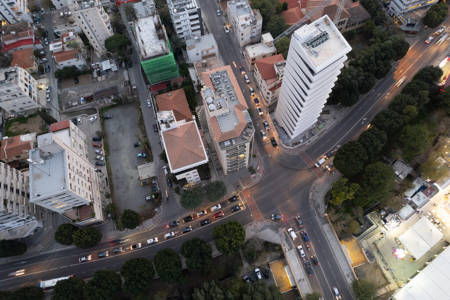
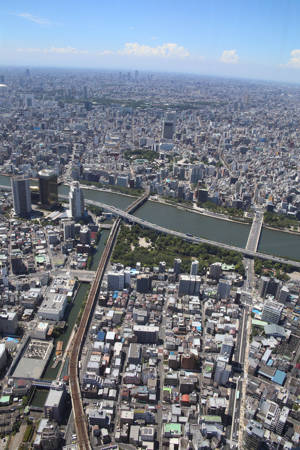
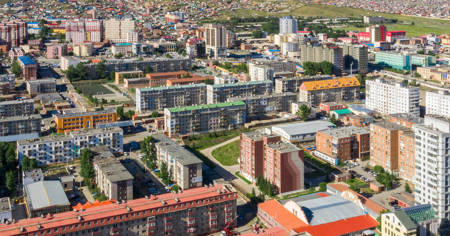
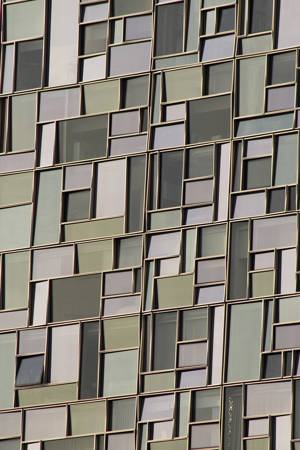
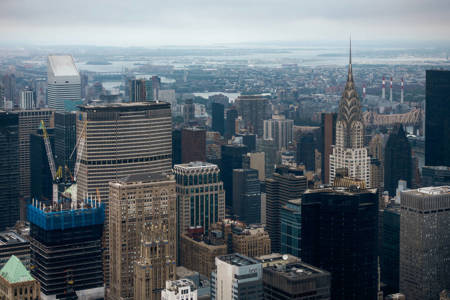
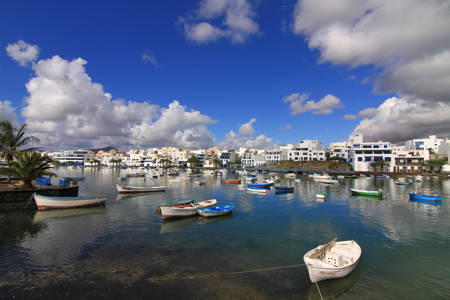
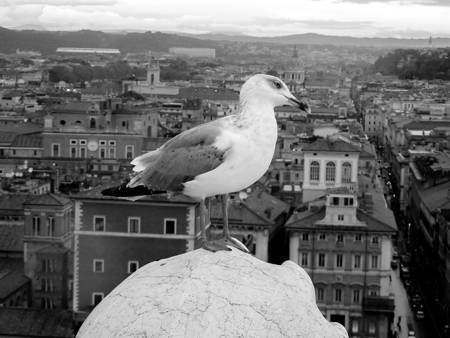
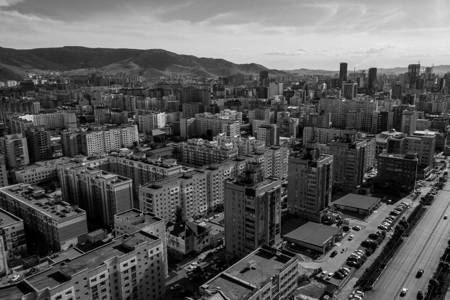
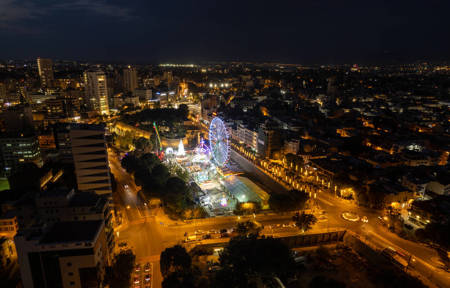
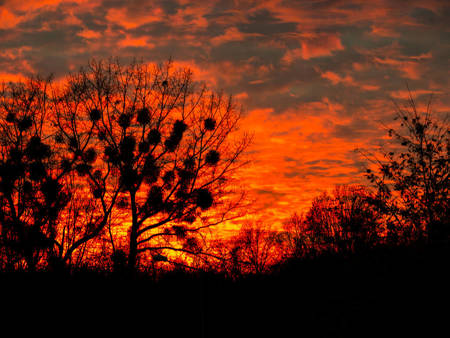
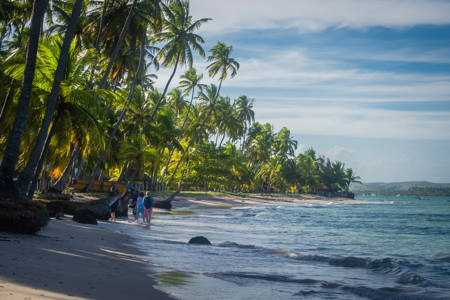
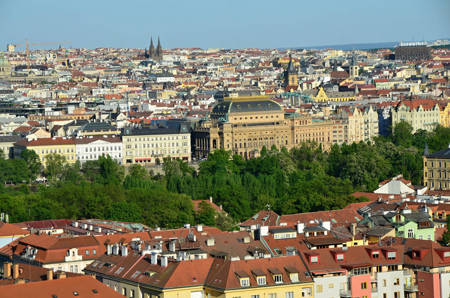
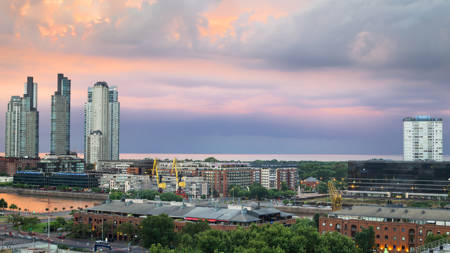
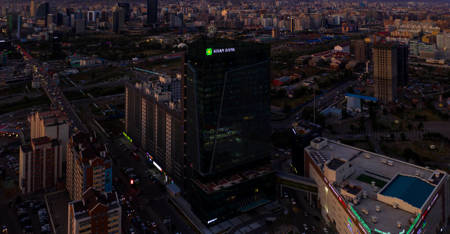
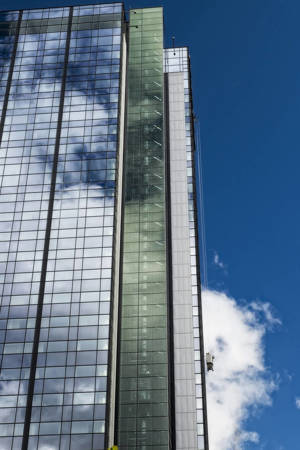
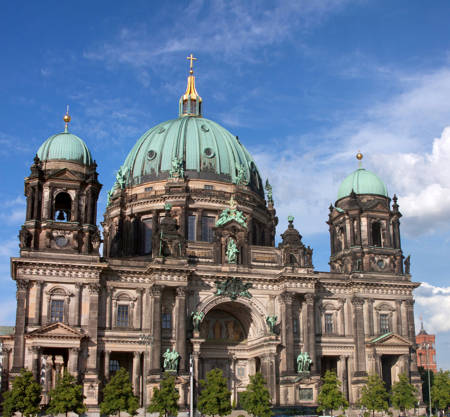
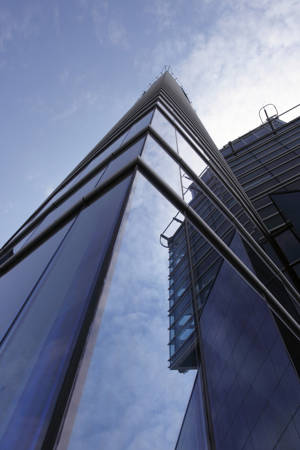
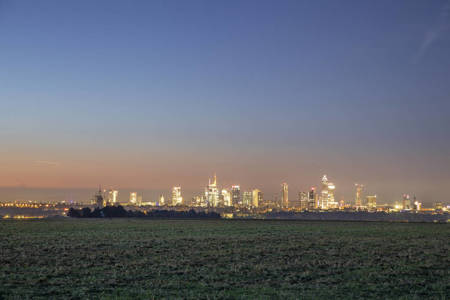
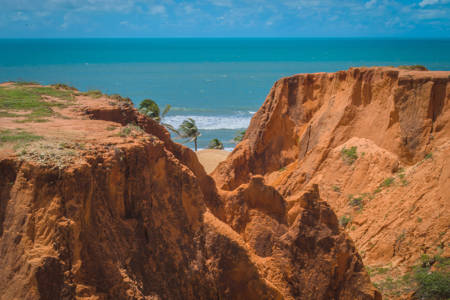
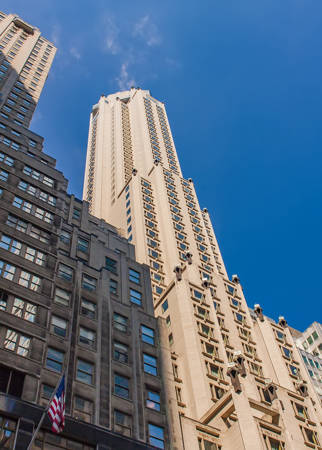
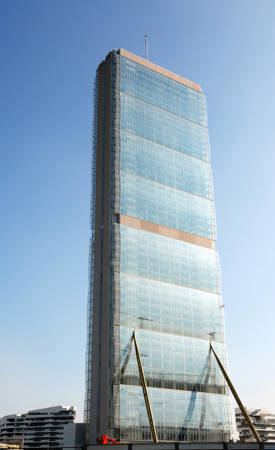
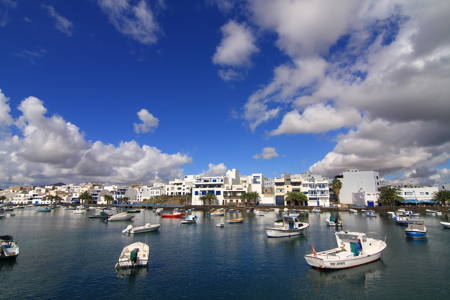
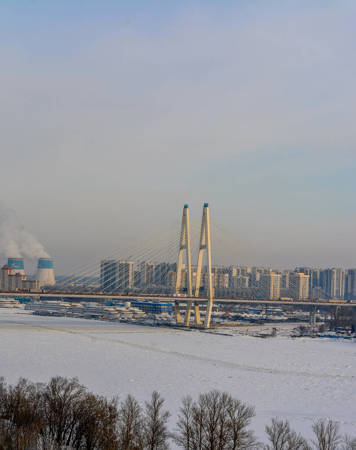
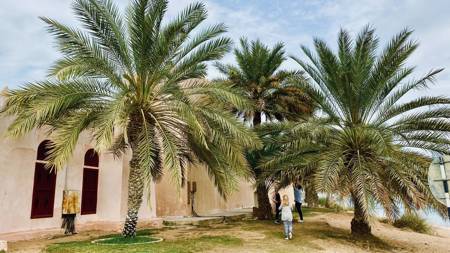
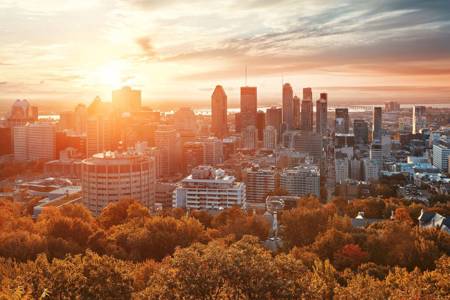
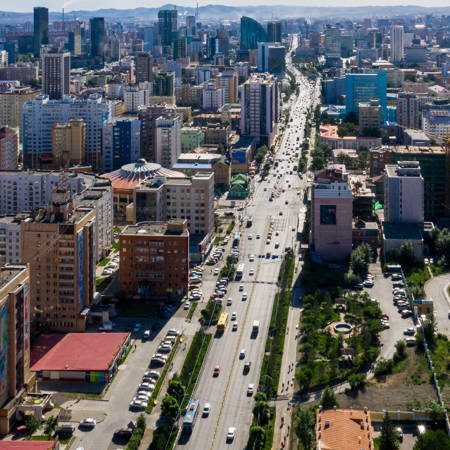
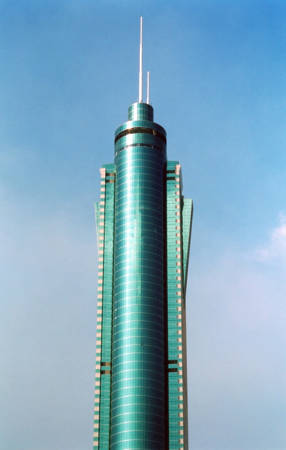
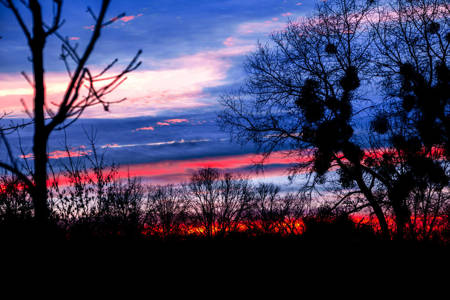
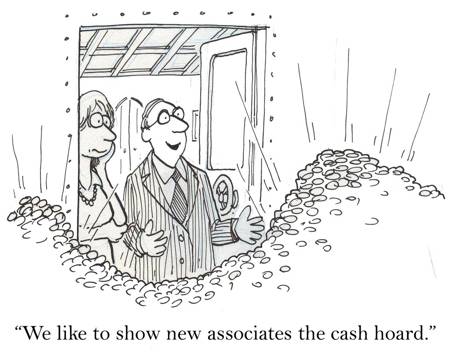
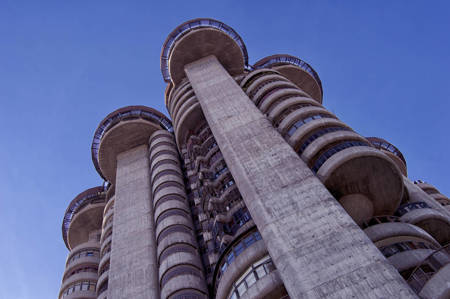
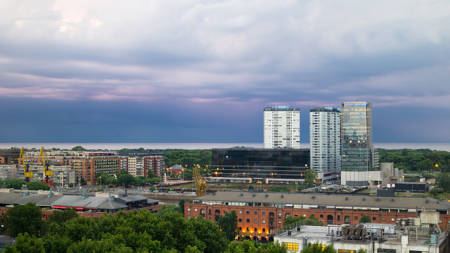
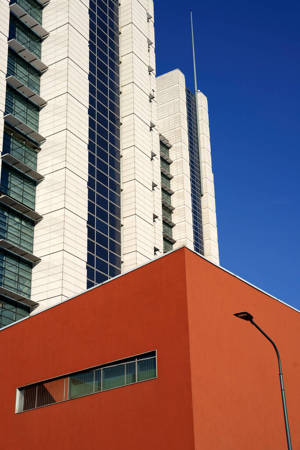
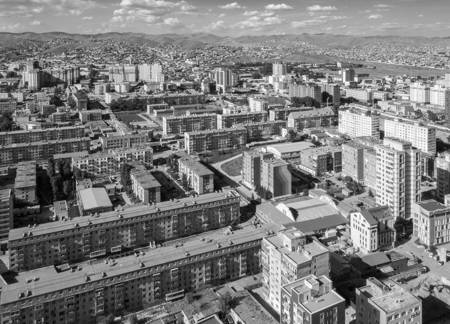