Evolving Narratives: Women's Multifaceted Impact in Varied Realms
Total images | : 9,583 |
Type | : organic |
Category | : Subjects |
Resolution | : Above 4K |
Storage size | : Up to 142 Gb |
File format | : JPEG |
The Women in Various Contexts dataset is a comprehensive collection that endeavors to shed light on the multifaceted roles, experiences, and contributions of women across diverse domains and situations. This meticulously collected dataset serves as a valuable resource for exploring and understanding the dynamic presence of women in a wide range of contexts, enabling researchers, policymakers, and enthusiasts to gain insights into the complexities of gender roles and societal dynamics.
This dataset holds immense potential for applications in the field of AI and machine learning, enabling the development of models that can uncover hidden patterns, predict trends, and contribute to advancements in gender studies. Some of the use cases in this domain include:
1. Gender Bias Detection in Media: Utilizing natural language processing (NLP) techniques, AI models can analyze media content from the dataset to identify and quantify gender biases in text, images, and videos. This insight can lead to more balanced and equitable media representations.
3. Healthcare Disparity Analysis: AI models can process healthcare-related data to identify disparities in access to medical services, diagnoses, and treatment options between genders. This information can inform healthcare policies and interventions.
4. Political Representation Forecasting: By analyzing historical data on women's representation in politics, AI models can predict future trends in political participation and suggest strategies to increase female representation in legislative bodies.
5. Gender-Neutral AI Language Models: The dataset can be used to train AI language models to generate gender-neutral text and reduce gender bias in automated content creation.
Environment: Commercial stock
Angle: Random
Augmentation: None
AR: Various
ACCURACY
This dataset contains a tolerance margin of 5% to 10% of associated images which might not reflect 100% accuracy in the metadata or image. As example for the error margin: a group photography, could feature also a male individual. All metadata in this dataset had been created manually and might contain a low margin of error. The maximum resolution of each image might vary.
128px
$89
256px
$179
512px
$269
1024px
$459
1920px
$699
Full Resolution
Contact
Sample images in this dataset
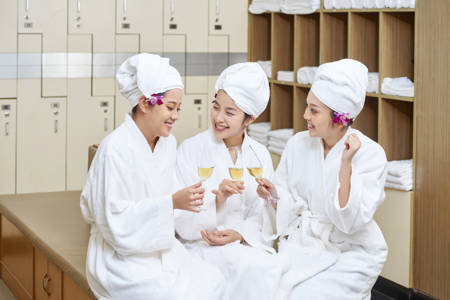
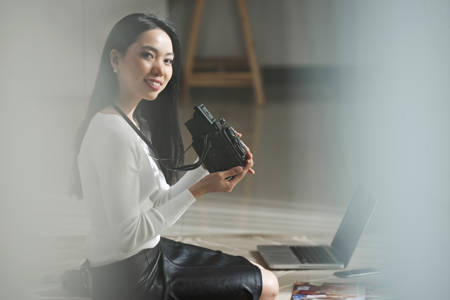
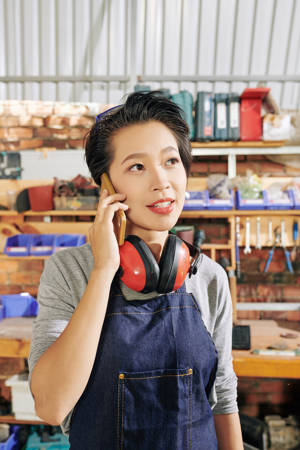
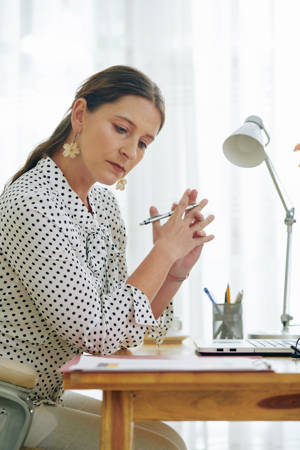
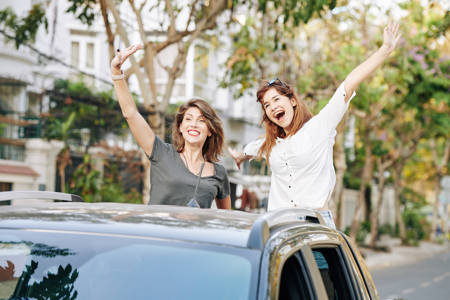
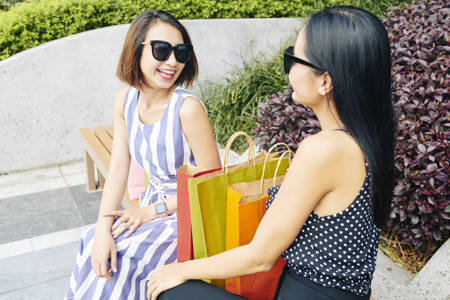
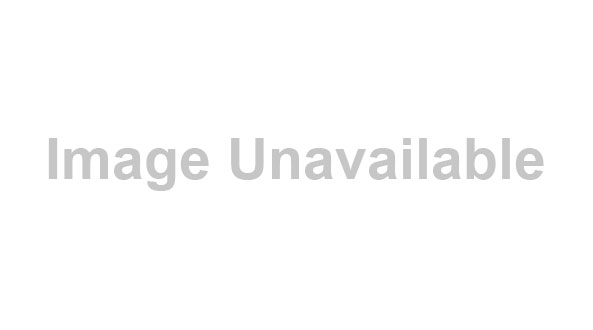
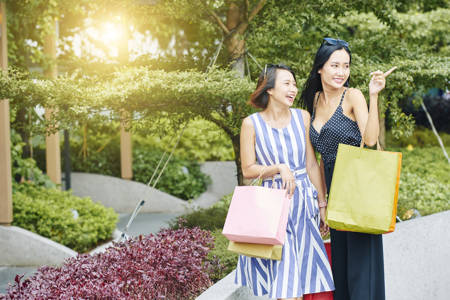
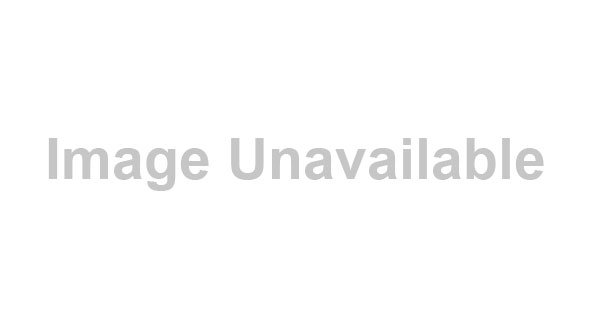
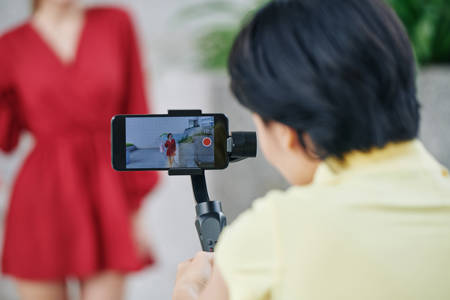
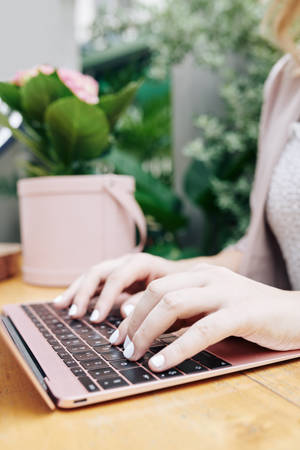
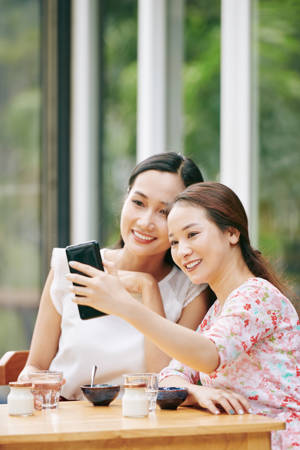
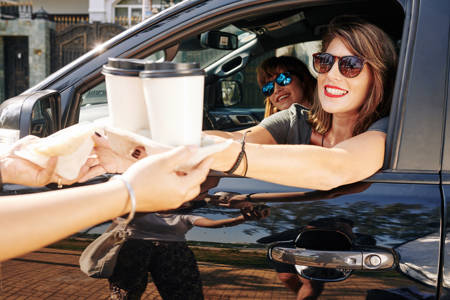
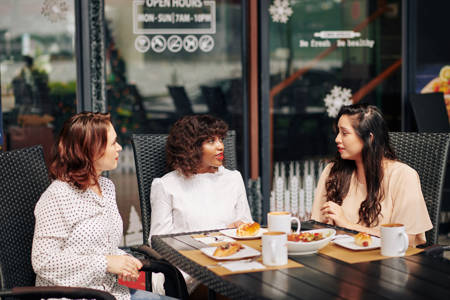
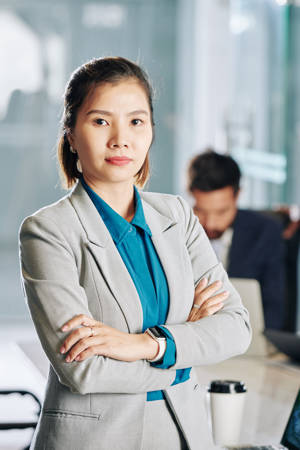
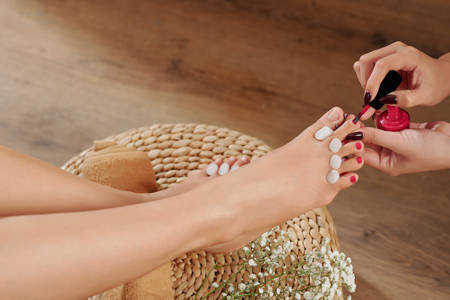
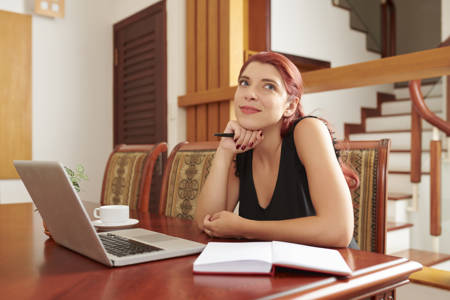
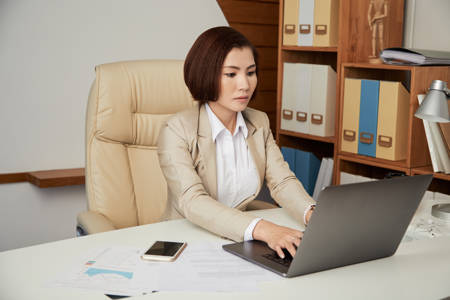
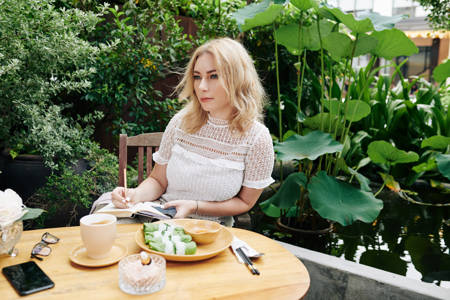
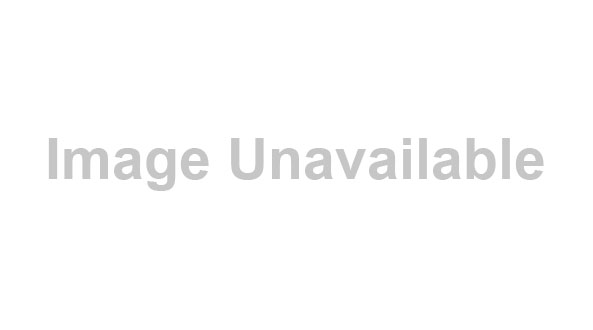
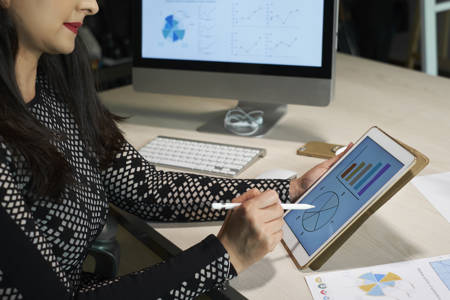
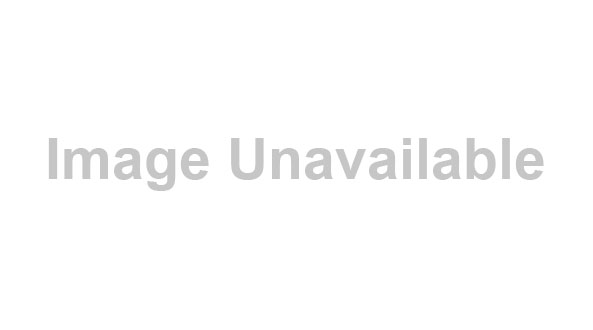
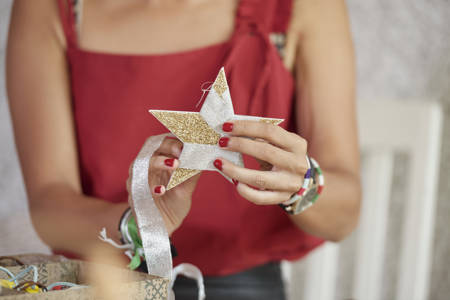
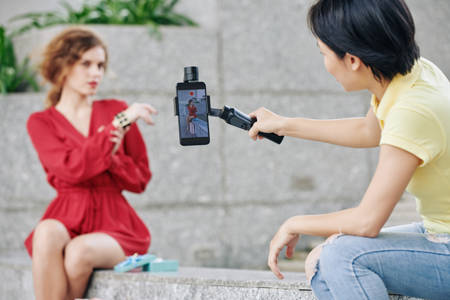
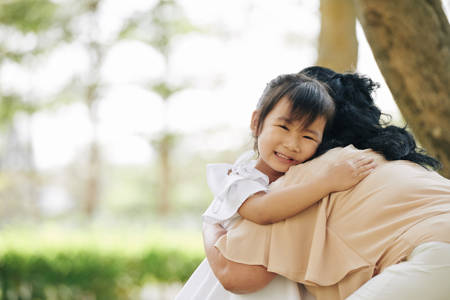
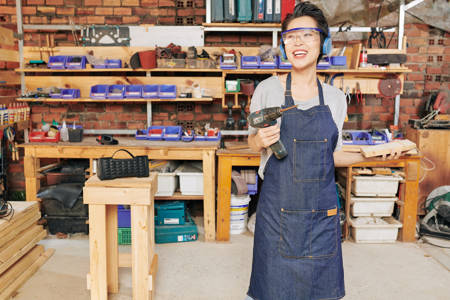
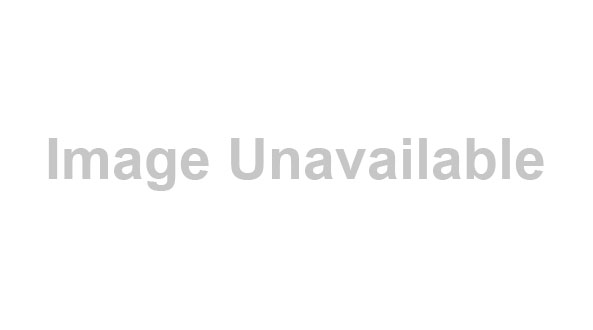
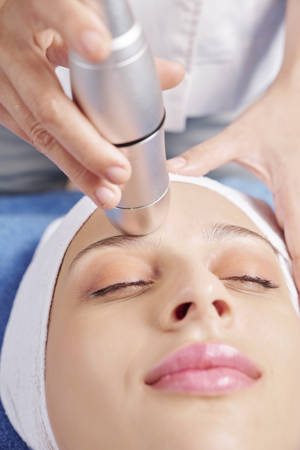
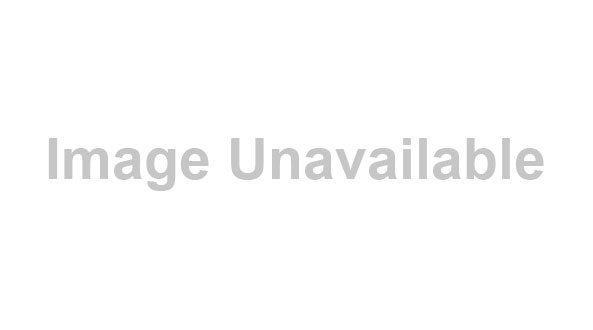
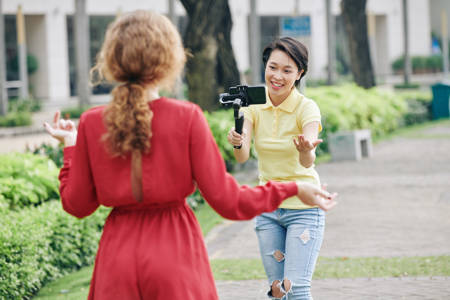
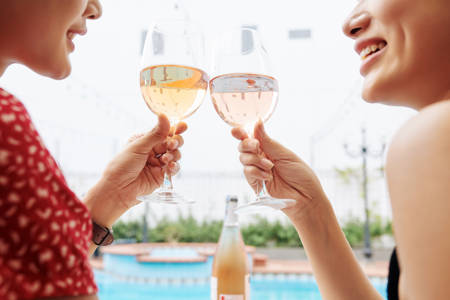
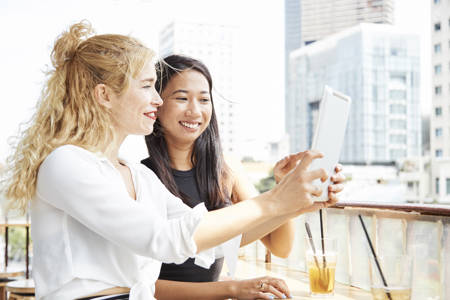
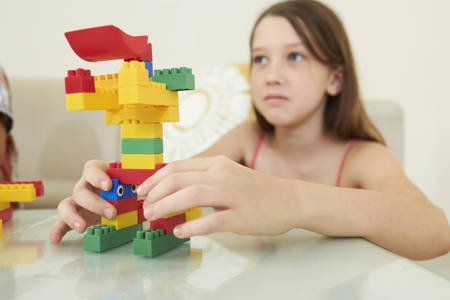
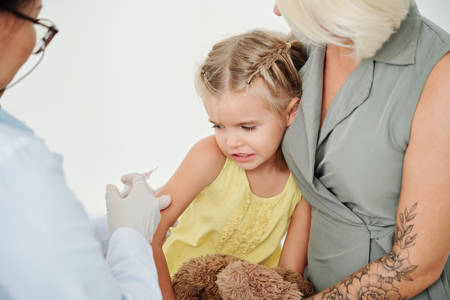
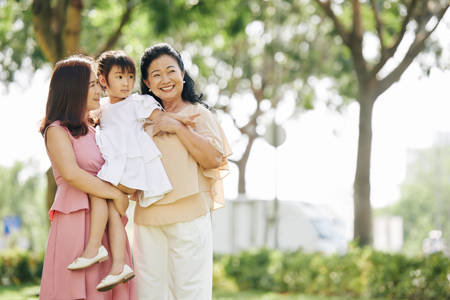
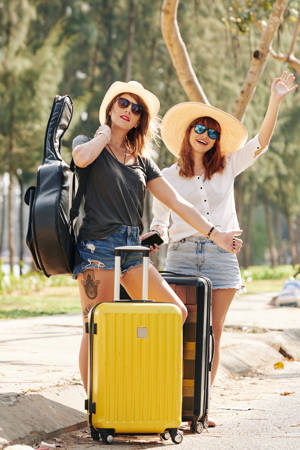
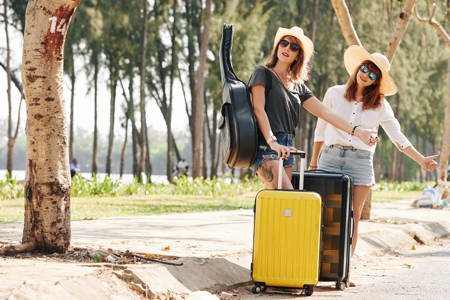
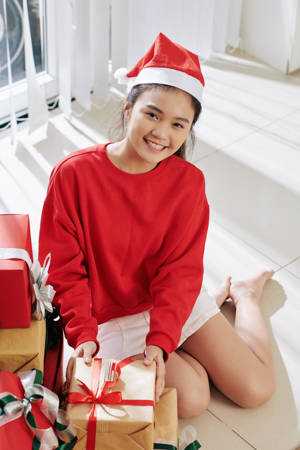
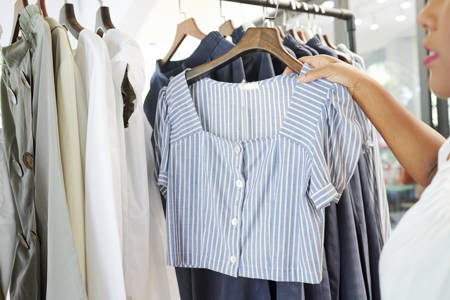
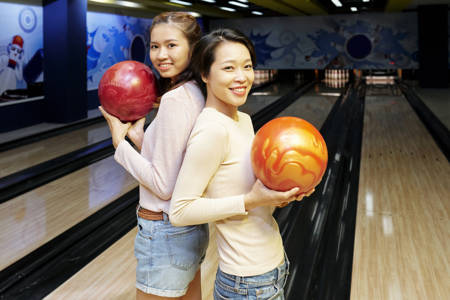
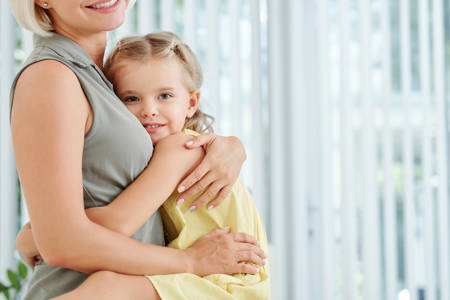
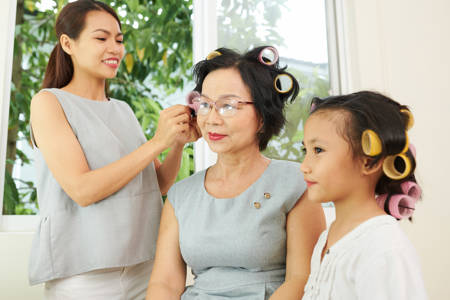
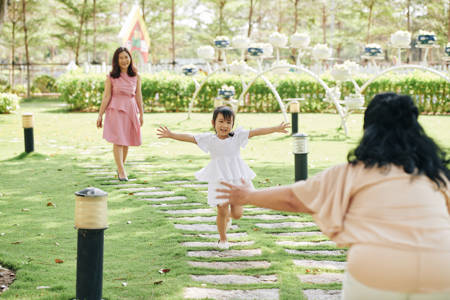
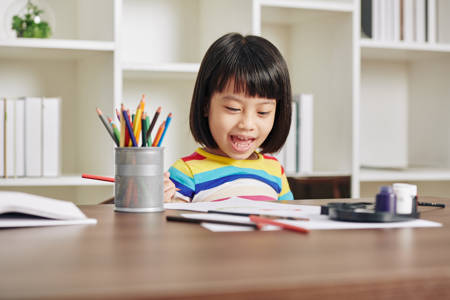
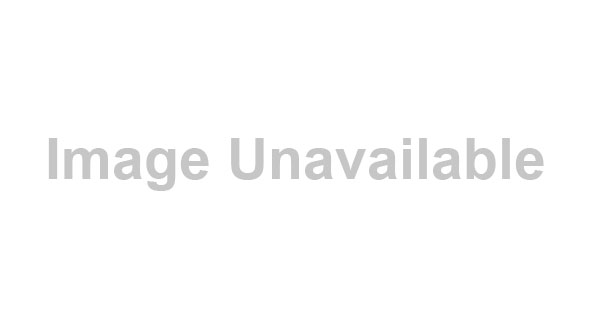