Dive into the World of Cycling Behaviors with Our Exclusive Dataset
Total samples | : 10 |
Type | : organic |
Category | : Subjects |
Resolution | : Above 4K |
File format | : JPEG |
The People in Various Cycling Contexts dataset is a comprehensive repository that offers a deep dive into the world of cycling, encompassing a wide range of cycling behaviors, environments, and contexts. This extensive collection serves as an invaluable resource for researchers, urban planners, transportation analysts, fitness enthusiasts, and AI developers seeking to gain insights into the multifaceted realm of cycling.
Empowered by cutting-edge AI and machine learning capabilities, this dataset provides a wealth of information for understanding cycling patterns, safety measures, and lifestyle preferences. Advanced algorithms allow researchers to uncover nuanced trends related to cycling activities, safety protocols, and the diverse contexts in which people ride bikes. Machine learning models enable predictive analysis of cycling trends, offering a forward-looking perspective on cycling behaviors and safety measures.
The People in Various Cycling Contexts dataset, enriched with AI and machine learning features, goes beyond traditional cycling databases, providing dynamic insights and predictive capabilities that redefine our understanding of cycling safety, urban planning, and the symbiotic relationship between cyclists and their environments. Its versatile applications include:
1. Cycling Behavior Analysis: Machine learning tools assist researchers in analyzing cycling behaviors, routes, and safety aspects, shedding light on factors like preferences, risks, and safety practices.
2. Urban Planning and Transportation: AI models contribute to the development of cycling-friendly infrastructure and transportation systems, making cities more accessible and sustainable for cyclists.
3. Fitness and Health Research: AI-driven evaluations help in assessing the health benefits of cycling and its impact on individuals and communities, promoting active lifestyles.
4. Cycling Safety Enhancement: Machine learning identifies safety trends and offers insights into measures to improve cycling safety, reducing accidents and injuries.
5. Transportation Policy Development: AI insights inform transportation policies, encouraging cycling as an eco-friendly mode of commuting and leisure.
6. Predictive Analytics: Machine learning models forecast future cycling trends, aiding in the planning of cycling-related initiatives and services.
Environment: Commercial stock
Angle: Random
Augmentation: None
AR: Various
ACCURACY
This dataset may contain images with a tolerance margin of 5% to 10% of associated images, which might not reflect 100% accuracy in the metadata or image content. For instance, an image of a cycling-related sign or road condition may be included due to its relevance to cycling behaviors. All metadata in this dataset has been created manually and might contain a low margin of error. The maximum resolution of each image might vary.
Register (no credit card required) to access your free dataset sample.
After registration, you can download this dataset and start exploring its contents.
Sample images in this dataset
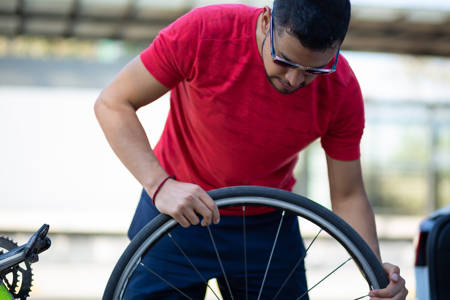
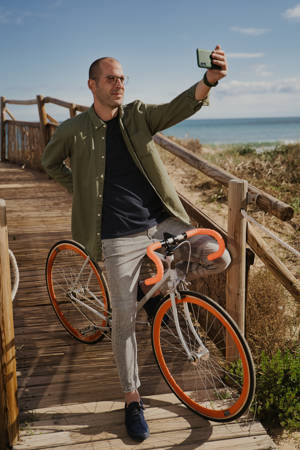
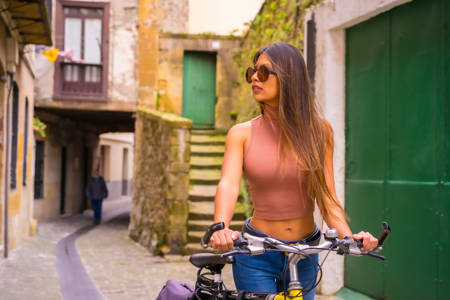
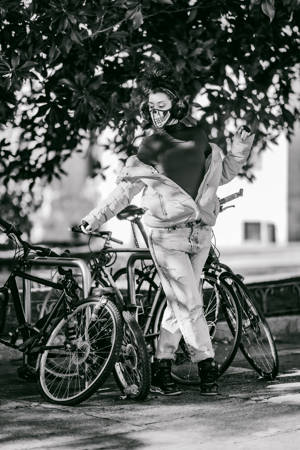
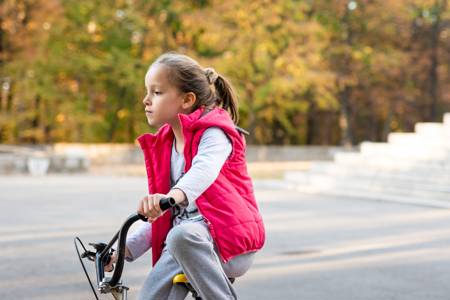
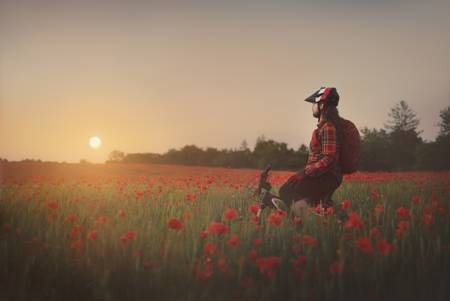
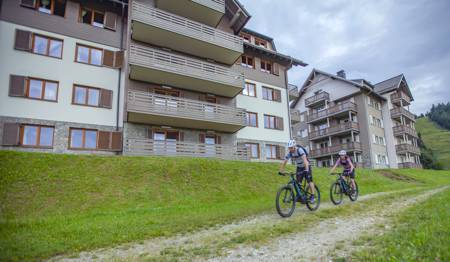
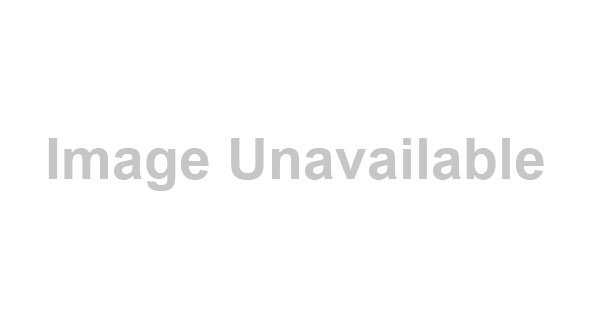